Titles for things are a concept that at one level seem very easy and straightforward. When you think of a book, it is usually pretty easy to identify the title of said book. When you start thinking about newspapers or other periodicals like magazines or journals, they are also usually pretty straightforward. You might think about The New York Times, or National Geographic, or maybe a local publication like the Denton Record-Chronicle. But when you start to work with many items in a library situation and have to represent the wide range of title variations, merging, splitting, publications with the same name, things get … messy.
Over the past 15 years, the UNT Libraries has approached managing serial and series titles in our digital collections by establishing two title qualifiers, serialtitle and seriestitle and then populating title fields using those qualifiers as appropriate. These qualified titles are then indexed so that they will show up in our facet lists and be searchable throughout the records as general metadata. We can also make use of them in situations when we want to identify a specific records with that title from the whole system. In our interfaces we highlight the existence of serial and series titles that occur more than once to try and lead our users to additional issues within a title.
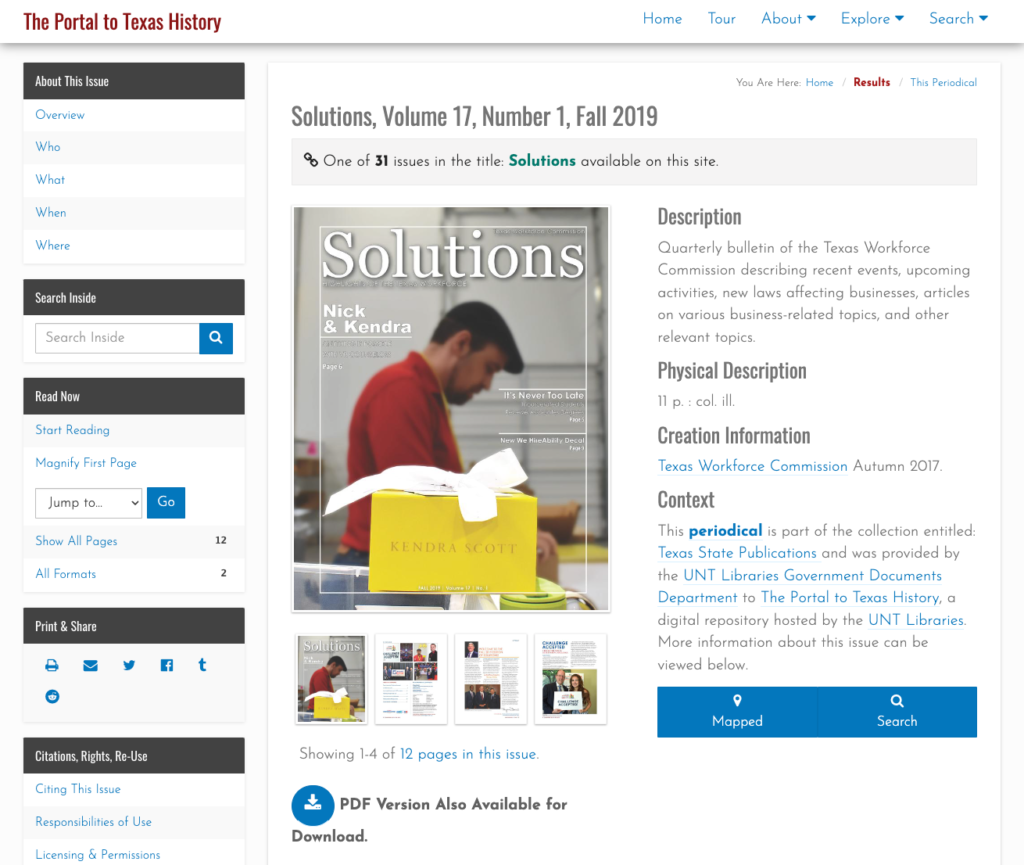
This actually works pretty well for many situations but there are a few things that cause it not to be so great. The first area this causes problems is when you have multiple publications that share the same name but that are in fact different publications. This happens somewhat often in the newspaper publishing world and since we have been trying to build large collections of newspapers from both Texas and Oklahoma, this has caused some challenges. One way of combatting this with newspapers specifically is to use a more unique version of the title. For example The Daily Herald was published in at least four different counties, Bexar, Cameron, Lamar, and Parker. In this case they represent four different titles though they share the same name. Instead of our current practice of putting The Daily Herald as the serial title we could have used The Daily Herald (Weatherford, Texas) or something similar to make it a bit clearer.
Another area that is challenging is the way that titles change over time. The example I gave at the beginning of this post of the Denton Record-Chronicle shows the merging of two different titles the Denton Record and the Denton Chronicle at some point in the past. These preceding, succeeding, or related titles are not easily managed with the simple system that we had in place in our system.
Finally when you want to link directly to a title in one of our digital library interfaces we are forced to link directly into our search interface, which results in URLs that people will include in other places that we are not going to be able to honor forever. An example of this is for the Solutions publication in the images above. Here is the resulting URL for this title in the system currently. https://texashistory.unt.edu/search/?fq=str_title_serial:%22Solutions%22
Titles as Things
Over the past year we have been working to untangle ourselves from our early title implementation. We wanted to design a system that would give us unique identifiers and URLs for each title that we are curating in our systems. We wanted to be able to connect titles together whenever appropriate. We wanted to be able to document metadata about the title itself like start and end years, place of publication, publisher, publishing frequency, language, and notate other identifiers like LCCN, OCLC, or ISSNs for a given title.
Another goal of the implementation was that we wanted to have a lightweight system that worked on top of existing records. We didn’t want to have to change over a million records to directly include this new title identifier in each metadata record. Instead we chose to work with existing OCLC and LCCN identifiers when they were available in records and directly include the title identifiers if there isn’t an OCLC or LCCN already created for the title.
With these ideas in mind we started to look at other implementations. We looked mainly at other large digital newspaper projects both in the US and around the world to see how they were approaching this space. We added a few requirements from this survey to our goals, primarily, the ability to have descriptions or essays about the title and the ability to further group titles into title families or other groupings.
We started our implementation with the base models from the Open Online Newspaper Initiative (Open ONI) which provided a great starting point. We also cribbed some features from the Georgia Historic Newspapers implementation as we thought they would be useful in our system.
We decided that each title would have an identifier minted with a t followed by a five digit, zero-padded number resulting in an identifier that looks like this t03303. To allow for the inclusion of these identifiers in records, we would introduce a new identifier qualifier, UNT-TITLE-ID in our UNTL Metadata Scheme.
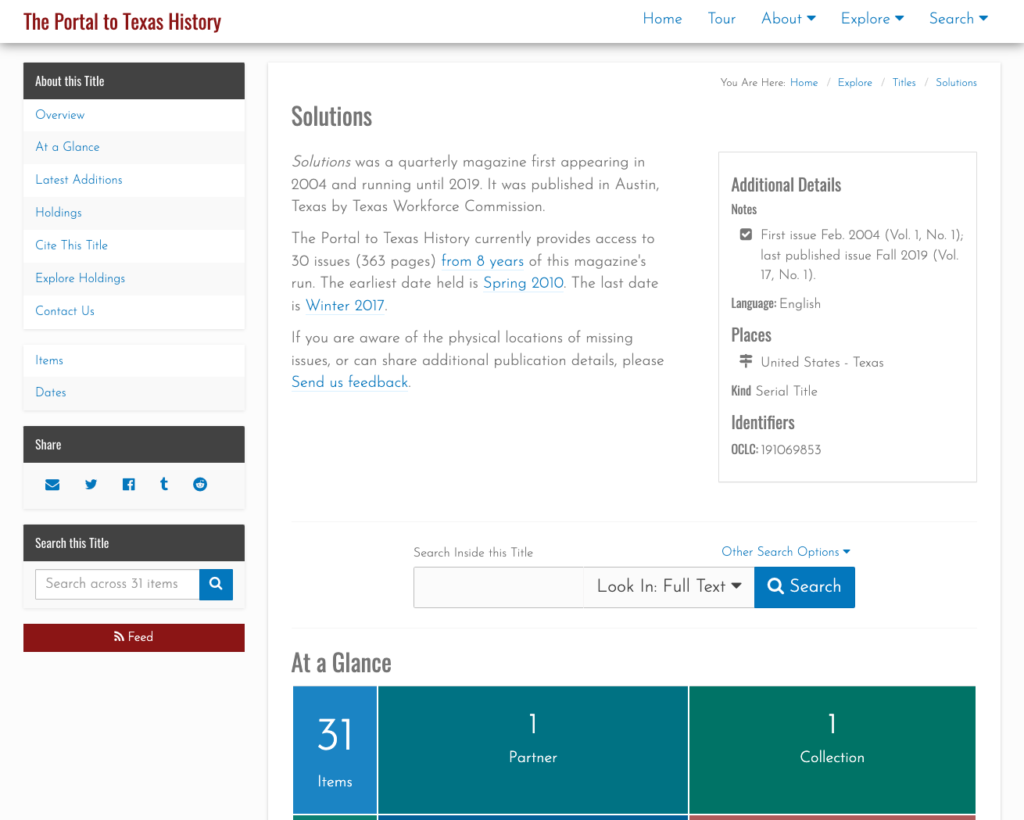
Now that we are able to create records for titles, the Solutions example that we used above has a Title Record in our system that we can link to and reference. In this case the URL is https://texashistory.unt.edu/explore/titles/t03303/
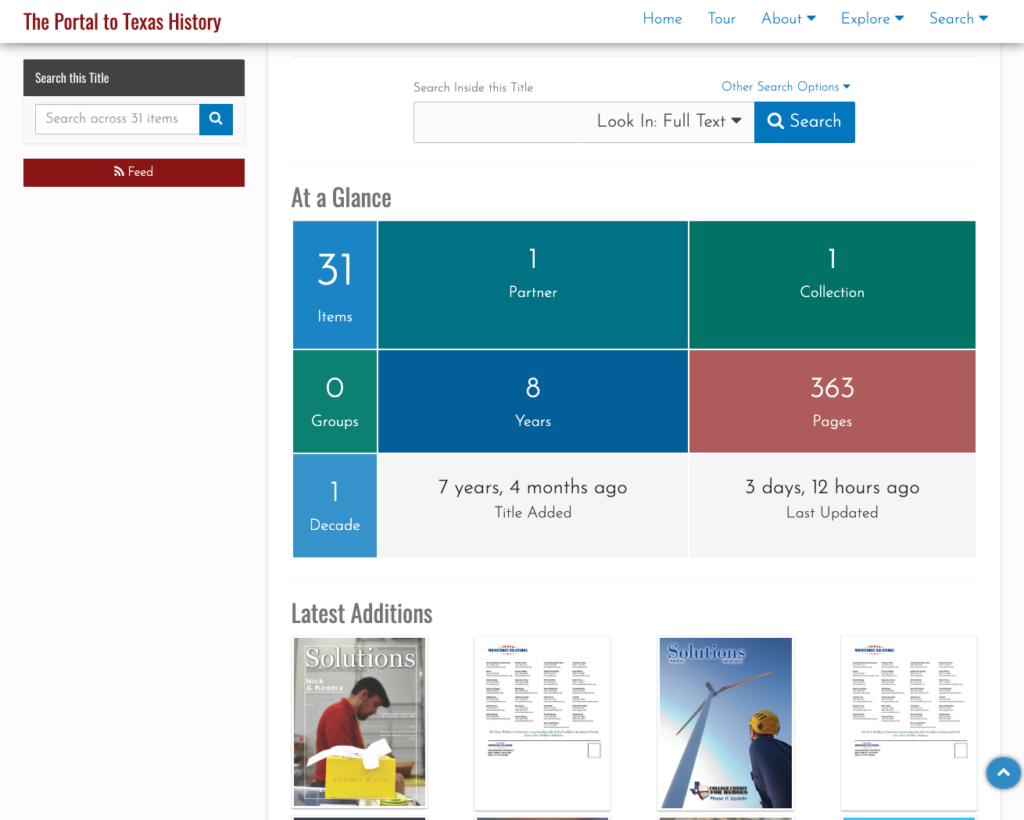
We tried to reference the design of the Collection and Partner pages in our new Title pages with the ability to search within a title and see an overview of some of the important metrics in the “At a Glance” section. Finally we show the most recently added issues of this publication in the “Latest Additions” section.
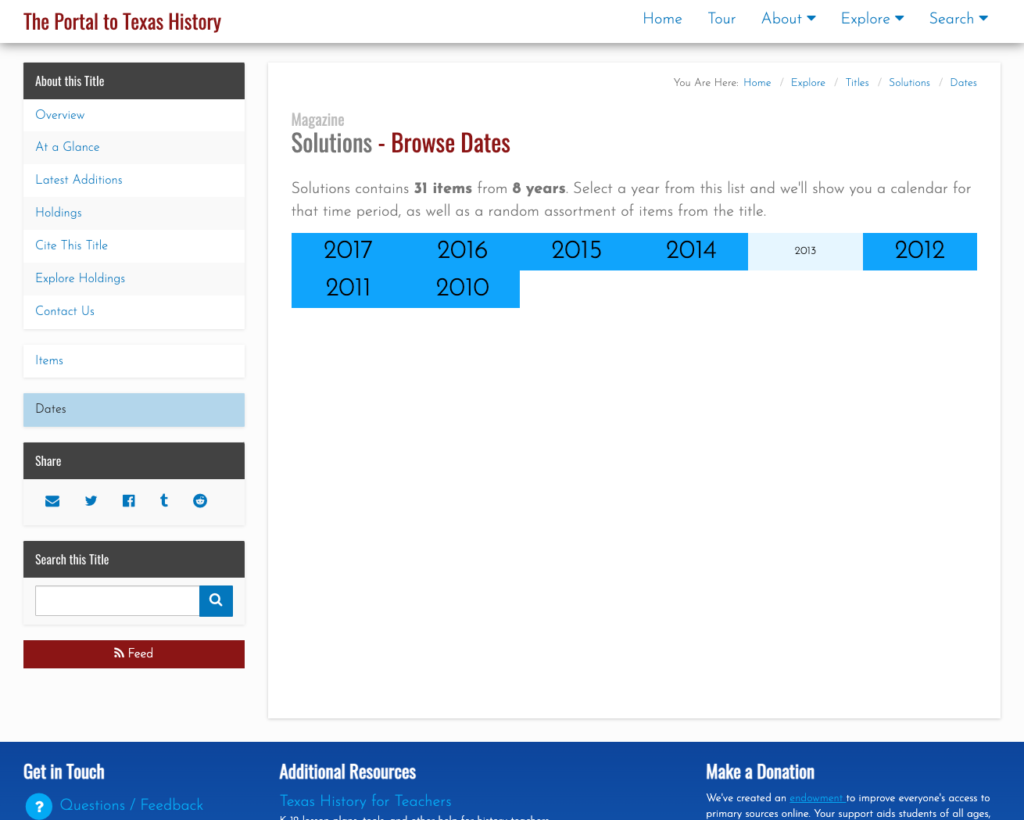
One of the more useful views that we are now able to provide is the “Dates” view. This allows a user to quickly see the years that are held for a publication. When a user clicks on a year they are given a calendar view so that they can select the month or date of the publication they are interested in viewing.

Finally, a users is able to view and search all of the issues of a title. This replicates the search interface and there are similar views in Collection and Partner pages that match this functionality. The full set of facets are available on the left to further drill into the content that the user is after.
Curated Titles
Now that we have a way of explicitly defining and describing titles in our systems we can pull those together into interfaces. The first page that we created was our curated title page (https://texashistory.unt.edu/explore/titles/curated/) which pulls together the currently available titles in one of our interfaces.
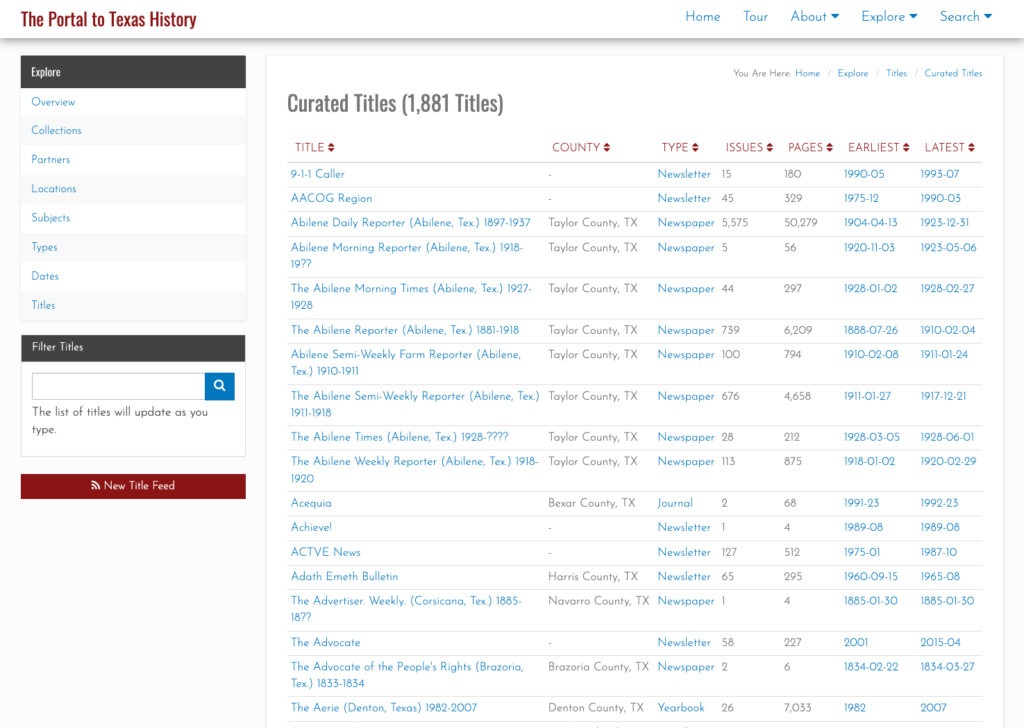
You can see the improvement for users who might be interested in looking at “The Daily Herald” like I mentioned at the top of this post. Now they are able to quickly distinguish between the different instances of this title and get to the one they are looking for.

All the other pieces.
I’ve presented a pretty high-level overview of the work that we have been doing for titles in The Portal to Texas History, the UNT Digital Library, and the Gateway to Oklahoma History. There are a ton of details that might be interesting such as the “not_in_database” helper that we created to identify titles that exist in the system but which do not have title record yet. We also completely overhauled the way we think about place names as part of this work, giving them their own models in a similar way as we did for the Titles. We also had to come up with a mechanism to represent holdings information so that we can quickly display that to users and systems when they request it from the titles interface. If there is interest in these topics I would be happy to write them up a bit.
If you have questions or comments about this post, please let me know via Twitter or Mastodon.